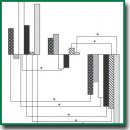
Higuchi Fractal Dimension as a Method for Assessing Response to Sound Stimuli in Patients with Diffuse Axonal Brain Injury
The aim of the research was to study the fractal dimension of the EEG signal by the Higuchi’s method in patients with diffuse axonal injury (DAI) of the brain.
Materials and Methods. The study was performed in 28 patients with DAI of different severity and 13 sex- and age-matched controls. The Higuchi’s method of fractal dimension was used to investigate brain response to sound stimuli of different emotional coloring as well as the features of the EEG signal in the resting state.
Results. The EEG data demonstrated the highest values of fractal dimension in patients with DAI in the resting state. The values of fractal dimension in different emotional states considerably differ both in healthy subjects and in those with DAI. An increase in fractal dimension in response to stimuli occurs predominantly at the frequency of the theta rhythm in the control group and the frequency of the alpha rhythm in the patients with severe DAI.
Conclusion. Higuchi fractal dimension can be used as a complementary diagnostic tool that allows differentiating perception of emotionally significant audio information in patients with brain injury.
- Pradhan N., Dutt D.N. Use of running fractal dimension for the analysis of changing patterns in electroencephalograms. Comput Biol Med 1993; 23(5): 381–388, https://doi.org/10.1016/0010-4825(93)90135-n.
- Raghavendra B.S., Dutt D.N. Signal characterization using fractal dimension. Fractals 2010; 18(3): 287–292, https://doi.org/10.1142/s0218348x10004968.
- Spasić S., Nikolić L., Mutavdžić D. Effect of a static magnetic field on the fractal complexity of bursting activity of the Br neuron in the snail detected by factor analysis. Arch Biol Sci 2011; 63(1): 177–183, https://doi.org/10.2298/abs1101177s.
- Lu U., Song D., Berger T.W. Nonlinear dynamic modeling of synaptically driven hippocampal neuron intracellular activity. IEEE Trans Biomed Eng 2011; 58(5): 1303–1313, https://doi.org/10.1109/tbme.2011.2105870.
- Arecchi F.T. Chaotic neuron dynamics, synchronization and feature binding. Physica A: Statistical Mechanics and its Applications 2004; 338(1–2): 218–237, https://doi.org/10.1016/j.physa.2004.02.045.
- Remme M.W.H., Lengyel M., Gutkin B.S. The role of ongoing dendritic oscillations in single-neuron dynamics. PLoS Comput Biol 2009; 5(9): e1000493, https://doi.org/10.1371/journal.pcbi.1000493.
- Higuchi T. Approach to an irregular time series on the basis of the fractal theory. Physica D: Nonlinear Phenomena 1988; 31(2): 277–283, https://doi.org/10.1016/0167-2789(88)90081-4.
- Acharya U.R., Faust O., Kannathal N., Chua T., Laxminarayan S. Non-linear analysis of EEG signals at various sleep stages. Comput Methods Programs Biomed 2005; 80(1): 37–45, https://doi.org/10.1016/j.cmpb.2005.06.011.
- Lin D.C., Sharif A., Kwan H.C. Scaling and organization of electroencephalographic background activity and alpha rhythm in healthy young adults. Biol Cybern 2006; 95(5): 401–411, https://doi.org/10.1007/s00422-006-0094-4.
- Zappasodi F., Olejarczyk E., Marzetti L., Assenza G., Pizzella V., Tecchio F. Fractal dimension of EEG activity senses neuronal impairment in acute stroke. PLoS One 2014; 9(6): e100199, https://doi.org/10.1371/journal.pone.0100199.
- Choong W.Y., Khairunizam W., Omar M.I., Murugappan M., Abdullah A.H., Ali H., Bong S.Z. EEG-based emotion assessment using detrended fluctuation analysis (DFA). Journal of Telecommunication, Electronic and Computer Engineering 2018; 10(1–13): 105–109.
- Päeske L., Bachmann M., Põld T., de Oliveira S.P.M., Lass J., Raik J., Hinrikus H. Surrogate data method requires end-matched segmentation of electroencephalographic signals to estimate non-linearity. Front Physiol 2018; 9: 1350, https://doi.org/10.3389/fphys.2018.01350.
- Spasić S., Kalauzi A., Ćulić M., Grbić G., Martać L. Estimation of parameter kmax in fractal analysis of rat brain activity. Ann N Y Acad Sci 2005; 1048(1): 427–429, https://doi.org/10.1196/annals.1342.054.
- Spasić S. Surrogate data test for nonlinearity of the rat cerebellar electrocorticogram in the model of brain injury. Signal Processing 2010; 90(12): 3015–3025, https://doi.org/10.1016/j.sigpro.2010.04.005.
- Kekovic G., Culic M., Martac L., Stojadinovic G., Capo I., Lalosevic D., Sekulic S. Fractal dimension values of cerebral and cerebellar activity in rats loaded with aluminium. Med Biol Eng Comput 2010; 48(7): 671–679, https://doi.org/10.1007/s11517-010-0620-3.
- Mayeux R., Ottman R., Maestre G., Ngai C., Tang M.X., Ginsberg H., Chun M., Tycko B., Shelanski M. Synergistic effects of traumatic head injury and apolipoprotein-epsilon4 in patients with Alzheimer’s disease. Neurology 1995; 45(3): 555–557, https://doi.org/10.1212/wnl.45.3.555.
- Wirth M., Bejanin A., La Joie R., Arenaza-Urquijo E.M., Gonneaud J., Landeau B., Perrotin A., Mézenge F., de La Sayette V., Desgranges B., Chételat G. Regional patterns of gray matter volume, hypometabolism, and beta-amyloid in groups at risk of Alzheimer’s disease. Neurobiol Aging 2018; 63: 140–151, https://doi.org/10.1016/j.neurobiolaging.2017.10.023.
- Stevenson R.A., James T.W. Affective auditory stimuli: characterization of the International Affective Digitized Sounds (IADS) by discrete emotional categories. Behav Res Methods 2008; 40(1): 315–321, https://doi.org/10.3758/brm.40.1.315.
- Delorme A., Makeig S. EEGLAB: an open source toolbox for analysis of single-trial EEG dynamics including independent component analysis. J Neurosci Methods 2004; 134(1): 9–21, https://doi.org/10.1016/j.jneumeth.2003.10.009.
- Klonowski W., Olejarczyk E., Stepienl R., Szelenberger W. New methods of nonlinear and symbolic dynamics in sleep EEG-signal analysis. IFAC Proceedings Volumes 2003; 36(15): 241–244, https://doi.org/10.1016/s1474-6670(17)33508-5.
- Šušmáková K., Krakovská A. Discrimination ability of individual measures used in sleep stages classification. Artif Intell Med 2008; 44(3): 261–277, https://doi.org/10.1016/j.artmed.2008.07.005.
- Ruiz-Padial E., Ibáñez-Molina A.J. Fractal dimension of EEG signals and heart dynamics in discrete emotional states. Biol Psychol 2018; 137: 42–48, https://doi.org/10.1016/j.biopsycho.2018.06.008.