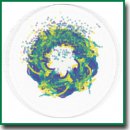
Radiomics in Determining Tumor-to-Normal Brain SUV Ratio Based on 11C-Methionine PET/CT in Glioblastoma
Modern methodology of PET/CT quantitative analysis in patients with glioblastomas is not strictly standardized in clinic settings and does not exclude the influence of the human factor. Methods of radiomics may facilitate unification, and improve objectivity and efficiency of the medical image analysis.
The aim of the study is to evaluate the potential of radiomics in the analysis of PET/CT glioblastoma images identifying the relationship between the radiomic features and the 11С-methionine tumor-to-normal brain uptake ratio (TNR) determined by an expert in routine.
Materials and Methods. PET/CT data (2018–2020) from 40 patients (average age was 55±12 years; 77.5% were males) with a histologically confirmed diagnosis of “glioblastoma” were included in the analysis. TNR was calculated as a ratio of the standardized uptake value of 11C-methionine measured in the tumor and intact tissue. Calculation of radiomic features for each PET was performed in the specified volumetric region of interest, capturing the tumor with the surrounding tissues. The relationship between TNR and the radiomic features was determined using the linear regression model. Predictors were included in the model following correlation analysis and LASSO regularization. The experiment with machine learning was repeated 300 times, splitting the training (70%) and test (30%) subsets randomly. The model quality metrics and predictor significance obtained in 300 tests were summarized.
Results. Of 412 PET/CT radiomic parameters significantly correlated with TNR (p<0.05), the regularization procedure left no more than 30 in each model (the median number of predictors was 9 [7; 13]). The experiment has demonstrated a non-random linear correlation (the Spearman correlation coefficient was 0.58 [0.43; 0.74]) between TNR and separate radiomic features, primarily fractal dimensions, characterizing the geometrical properties of the image.
Conclusion. Radiomics enabled an objective determination of PET/CT image texture features reflecting the biological activity of glioblastomas. Despite the existing limitations in the application, the first results provide a good perspective of these methods in neurooncology.
- Ostrom Q.T., Cioffi G., Waite K., Kruchko C., Barnholtz-Sloan J.S. CBTRUS statistical report: primary brain and other central nervous system tumors diagnosed in the United States in 2014–2018. Neuro Oncol 2021; 23(12 Suppl 2): iii1–iii105, https://doi.org/10.1093/neuonc/noab200.
- Nikitin P.V., Ryzhova M.V., Zubova I.V., Panina T.N., Shugay S.V. Heterogeneity of tumor cells in glioblastomas. Arhiv patologii 2019; 81(3): 27–36, https://doi.org/10.17116/patol20198103127.
- Vikhrova N.B., Kalaeva D.B., Batalov A.I., Konakova T.A., Khokhlova E.V., Postnov A.A., Pronin I.N. 11C-methionine and 18F-fluorodeoxyglucose PET/CT in diagnosis of brain gliomas heterogeneity. Rossijskij elektronnyj zurnal lucevoj diagnostiki 2021; 11(2): 68–82.
- Litvin A.A., Burkin D.A., Kropinov A.A., Paramzin F.N. Radiomics and digital image texture analysis in oncology (review). Sovremennye tehnologii v medicine 2021; 13(2): 97, https://doi.org/10.17691/stm2021.13.2.11.
- Kocher M., Ruge M.I., Galldiks N., Lohmann P. Applications of radiomics and machine learning for radiotherapy of malignant brain tumors. Strahlenther Onkol 2020; 196(10): 856–867, https://doi.org/10.1007/s00066-020-01626-8.
- Danilov G.V., Shifrin M.A., Kotik K.V., Ishankulov T.A., Orlov Yu.N., Kulikov A.S., Potapov A.A. Artificial intelligence in neurosurgery: a systematic review using topic modeling. Part I: major research areas. Sovremennye tehnologii v medicine 2020; 12(5): 106, https://doi.org/10.17691/stm2020.12.5.12.
- Lambin P., Leijenaar R.T.H., Deist T.M., Peerlings J., de Jong E.E.C., van Timmeren J., Sanduleanu S., Larue R.T.H.M., Even A.J.G., Jochems A., van Wijk Y., Woodruff H., van Soest J., Lustberg T., Roelofs E., van Elmpt W., Dekker A., Mottaghy F.M., Wildberger J.E., Walsh S. Radiomics: the bridge between medical imaging and personalized medicine. Nat Rev Clin Oncol 2017; 14(12): 749–762, https://doi.org/10.1038/nrclinonc.2017.141.
- Zhu M., Li S., Kuang Y., Hill V.B., Heimberger A.B., Zhai L., Zhai S. Artificial intelligence in the radiomic analysis of glioblastomas: a review, taxonomy, and perspective. Front Oncol 2022; 12: 924245, https://doi.org/10.3389/fonc.2022.924245.
- Kolossváry M. RIA package manual. URL: https://cran.r-project.org/web/packages/RIA/vignettes/RIA.html.
- Kolossváry M., Karády J., Szilveszter B., Kitslaar P., Hoffmann U., Merkely B., Maurovich-Horvat P. Radiomic features are superior to conventional quantitative computed tomographic metrics to identify coronary plaques with napkin-ring sign. Circ Cardiovasc Imaging 2017; 10(12): e006843, https://doi.org/10.1161/circimaging.117.006843.
- Cao X., Tan D., Liu Z., Liao M., Kan Y., Yao R., Zhang L., Nie L., Liao R., Chen S., Xie M. Differentiating solitary brain metastases from glioblastoma by radiomics features derived from MRI and 18F-FDG-PET and the combined application of multiple models. Sci Rep 2022; 12(1): 5722, https://doi.org/10.1038/s41598-022-09803-8.
- Zhang L., Yao R., Gao J., Tan D., Yang X., Wen M., Wang J., Xie X., Liao R., Tang Y., Chen S., Li Y. An integrated radiomics model incorporating diffusion-weighted imaging and 18F-FDG PET imaging improves the performance of differentiating glioblastoma from solitary brain metastases. Front Oncol 2021; 11: 732704, https://doi.org/10.3389/fonc.2021.732704.
- Li Z., Holzgreve A., Unterrainer L.M., Ruf V.C., Quach S., Bartos L.M., Suchorska B., Niyazi M., Wenter V., Herms J., Bartenstein P., Tonn J.C., Unterrainer M., Albert N.L., Kaiser L. Combination of pre-treatment dynamic [18F]FET PET radiomics and conventional clinical parameters for the survival stratification in patients with IDH-wildtype glioblastoma. Eur J Nucl Med Mol Imaging 2022, https://doi.org/10.1007/s00259-022-05988-2.
- Barry N., Rowshanfarzad P., Francis R.J., Nowak A.K., Ebert M.A. Repeatability of image features extracted from FET PET in application to post-surgical glioblastoma assessment. Phys Eng Sci Med 2021; 44(4): 1131–1140, https://doi.org/10.1007/s13246-021-01049-4.
- Carles M., Popp I., Starke M.M., Mix M., Urbach H., Schimek-Jasch T., Eckert F., Niyazi M., Baltas D., Grosu A.L. FET-PET radiomics in recurrent glioblastoma: prognostic value for outcome after re-irradiation? Radiat Oncol 2021; 16(1): 46, https://doi.org/10.1186/s13014-020-01744-8.
- Lohmann P., Elahmadawy M.A., Gutsche R., Werner J.M., Bauer E.K., Ceccon G., Kocher M., Lerche C.W., Rapp M., Fink G.R., Shah N.J., Langen K.J., Galldiks N. FET PET radiomics for differentiating pseudoprogression from early tumor progression in glioma patients post-chemoradiation. Cancers (Basel) 2020; 12(12): 3835, https://doi.org/10.3390/cancers12123835.
- Kong Z., Jiang C., Zhu R., Feng S., Wang Y., Li J., Chen W., Liu P., Zhao D., Ma W., Wang Y., Cheng X. 18F-FDG-PET-based radiomics features to distinguish primary central nervous system lymphoma from glioblastoma. Neuroimage (Amst) 2019; 23: 101912, https://doi.org/10.1016/j.nicl.2019.101912.
- Qian J., Herman M.G., Brinkmann D.H., Laack N.N., Kemp B.J., Hunt C.H., Lowe V., Pafundi D.H. Prediction of MGMT status for glioblastoma patients using radiomics feature extraction from 18F-DOPA-PET imaging. Int J Radiat Oncol Biol Phys 2020; 108(5): 1339–1346, https://doi.org/10.1016/j.ijrobp.2020.06.073.