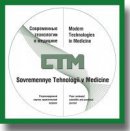
Нейрогенетика функциональной коннективности мозга: современные подходы к изучению (обзор)
Благодаря развитию техник нейровизуализации выделен ряд функциональных сетей мозга, связанных как с конкретными функциями, так и с состоянием относительного бездействия. Накоплено достаточно информации об изменениях коннективности (связей между областями мозга) при психопатологиях, например депрессии, шизофрении, аутизме. Ведутся активные исследования их генетических маркеров, для чего используется поиск генов-кандидатов, а также полногеномный поиск генетических ассоциаций. При этом данных, рассматривающих коннективность как промежуточное звено в цепочке генотип–патология, не так много, хотя она представляется надежным эндофенотипом, поскольку демонстрирует высокую стабильность и высокую наследуемость. В данном обзоре рассматриваются результаты исследований, посвященных поиску биомаркеров, молекулярно-генетических ассоциаций функциональной, а также частично анатомической и эффективной коннективности. Описаны основные подходы к оценке нейрогенетики коннективности, а также конкретные генетические варианты, для которых была обнаружена связь с коннективностью мозга при психических патологиях.
- Kasthuri N., Hayworth K.J., Berger D.R., Schalek R.L., Conchello J.A., Knowles-Barley S., Lee D., Vázquez-Reina A., Kaynig V., Jones T.R., Roberts M., Morgan J.L., Tapia J.C., Seung H.S., Roncal W.G., Vogelstein J.T., Burns R., Sussman D.L., Priebe C.E., Pfister H., Lichtman J.W. Saturated reconstruction of a volume of neocortex. Cell 2015; 162(3): 648–661, https://doi.org/10.1016/j.cell.2015.06.054.
- Aertsen A.M., Gerstein G.L., Habib M.K., Palm G. Dynamics of neuronal firing correlation: modulation of “effective connectivity”. J Neurophysiol 1989; 61(5): 900–917, https://doi.org/10.1152/jn.1989.61.5.900.
- Friston K.J., Frith C.D., Liddle P.F., Frackowiak R.S.J. Functional connectivity: the principal-component analysis of large (PET) data sets. J Cereb Blood Flow Metab 1993; 13: 5–14, https://doi.org/10.1038/jcbfm.1993.4.
- Power J.D., Cohen A.L., Nelson S.M., Wig G.S., Barnes K.A., Church J.A., Vogel A.C., Laumann T.O., Miezin F.M., Schlaggar B.L., Petersen S.E. Functional network organization of the human brain. Neuron 2011; 72(4): 665–678, https://doi.org/10.1016/j.neuron.2011.09.006.
- Honey C.J., Sporns O., Cammoun L., Gigandet X., Thiran J.P., Meuli R., Hagmann P. Predicting human resting-state functional connectivity from structural connectivity. Proc Natl Acad Sci U S A 2009; 106(6): 2035–2040, https://doi.org/10.1073/pnas.0811168106.
- Mesulam M.M. From sensation to cognition. Brain 1998; 121(6): 1013–1052, https://doi.org/10.1093/brain/121.6.1013.
- Friston K.J. Functional and effective connectivity: a review. Brain Connect 2011; 1(1): 13–36, https://doi.org/10.1089/brain.2011.0008.
- Corbetta M., Kincade J.M., Shulman G.L. Neural systems for visual orienting and their relationships to spatial working memory. J Cogn Neurosci 2002; 14(3): 508–523, https://doi.org/10.1162/089892902317362029.
- Fox M.D., Snyder A.Z., Vincent J.L., Corbetta M., Van Essen D.C., Raichle M.E. The human brain is intrinsically organized into dynamic, anticorrelated functional networks. Proc Natl Acad Sci U S A 2005; 102(27): 9673–9678, https://doi.org/10.1073/pnas.0504136102.
- Martynova O.V., Balaev V.V. Age related changes in functional connectivity of the resting state networks. Psychol J High Sch Econ 2015; 12(4): 33–47.
- Meyer-Lindenberg A. The future of fMRI and genetics research. Neuroimage 2012; 62(2): 1286–1292, https://doi.org/10.1016/j.neuroimage.2011.10.063.
- Barch D.M., Burgess G.C., Harms M.P., Petersen S.E., Schlaggar B.L., Corbetta M., Glasser M.F., Curtiss S., Dixit S., Feldt C., Nolan D., Bryant E., Hartley T., Footer O., Bjork J.M., Poldrack R., Smith S., Johansen-Berg H., Snyder A.Z., Van Essen D.C.; WU-Minn HCP Consortium. Function in the human connectome: task-fMRI and individual differences in behavior. Neuroimage 2013; 80: 169–189, https://doi.org/10.1016/j.neuroimage.2013.05.033.
- Finn E.S., Shen X., Scheinost D., Rosenberg M.D., Huang J., Chun M.M., Papademetris X., Constable R.T. Functional connectome fingerprinting: identifying individuals using patterns of brain connectivity. Nat Neurosci 2015; 18(11): 1664–1671, https://doi.org/10.1038/nn.4135.
- Perry A., Roberts G., Mitchell P.B., Breakspear M. Connectomics of bipolar disorder: a critical review, and evidence for dynamic instabilities within interoceptive networks. Mol Psychiatry 2019; 24(9): 1296–1318, https://doi.org/10.1038/s41380-018-0267-2.
- Wales R.M., Leung H.C. The effects of amyloid and tau on functional network connectivity in older populations. Brain Connect 2021; 11(8): 599–612, https://doi.org/10.1089/brain.2020.0902.
- Kochunov P., Glahn D.C., Lancaster J.L., Winkler A.M., Smith S., Thompson P.M., Almasy L., Duggirala R., Fox P.T., Blangero J. Genetics of microstructure of cerebral white matter using diffusion tensor imaging. Neuroimage 2010; 53(3): 1109–1116, https://doi.org/10.1016/j.neuroimage.2010.01.078.
- Jahanshad N., Hibar D.P., Ryles A., Toga A.W., McMahon K.L., de Zubicaray G.I., Hansell N.K., Montgomery G.W., Martin N.G., Wright M.J., Thompson P.M. Discovery of genes that affect human brain connectivity: a genome-wide analysis of the connectome. In: Proc IEEE Int Symp Biomed Imaging. Barcelona; 2012; p. 542–545, https://doi.org/10.1109/isbi.2012.6235605.
- Thompson P.M., Ge T., Glahn D.C., Jahanshad N., Nichols T.E. Genetics of the connectome. Neuroimage 2013; 80: 475–488, https://doi.org/10.1016/j.neuroimage.2013.05.013.
- Jakab A., Emri M., Spisak T., Szeman-Nagy A., Beres M., Kis S.A., Molnar P., Berenyi E. Autistic traits in neurotypical adults: correlates of graph theoretical functional network topology and white matter anisotropy patterns. PLoS One 2013; 8(4): e60982, https://doi.org/10.1371/journal.pone.0060982.
- White T., Gottesman I. Brain connectivity and gyrification as endophenotypes for schizophrenia: weight of the evidence. Curr Top Med Chem 2012; 12(21): 2393–2403, https://doi.org/10.2174/156802612805289953.
- Glahn D.C., Winkler A.M., Kochunov P., Almasy L., Duggirala R., Carless M.A., Curran J.C., Olvera R.L., Laird A.R., Smith S.M., Beckmann C.F., Fox P.T., Blangero J. Genetic control over the resting brain. Proc Natl Acad Sci U S A 2010; 107(3): 1223–1228, https://doi.org/10.1073/pnas.0909969107.
- Collin G., Kahn R.S., de Reus M.A., Cahn W., van den Heuvel M.P. Impaired rich club connectivity in unaffected siblings of schizophrenia patients. Schizophr Bull 2014; 40(2): 438–448, https://doi.org/10.1093/schbul/sbt162.
- Doll C.A., Broadie K. Impaired activity-dependent neural circuit assembly and refinement in autism spectrum disorder genetic models. Front Cell Neurosci 2014; 8: 30, https://doi.org/10.3389/fncel.2014.00030.
- Fornito A., Zalesky A., Bassett D.S., Meunier D., Ellison-Wright I., Yücel M., Wood S.J., Shaw K., O’Connor J., Nertney D., Mowry B.J., Pantelis C., Bullmore E.T. Genetic influences on cost-efficient organization of human cortical functional networks. J Neurosci 2011; 31(9): 3261–3270, https://doi.org/10.1523/jneurosci.4858-10.2011.
- Su T.P., Chen M.H., Tu P.C. Using big data of genetics, health claims, and brain imaging to challenge the categorical classification in mental illness. J Chin Med Assoc 2022; 85(2): 139–144, https://doi.org/10.1097/jcma.0000000000000675.
- Foo H., Thalamuthu A., Jiang J., Koch F.C., Mather K.A., Wen W., Sachdev P.S. Novel genetic variants associated with brain functional networks in 18,445 adults from the UK Biobank. Sci Rep 2021; 11(1): 14633, https://doi.org/10.1038/s41598-021-94182-9.
- Bullmore E., Sporns O. Complex brain networks: graph theoretical analysis of structural and functional systems. Nat Rev Neurosci 2009; 10(3): 186–198, https://doi.org/10.1038/nrn2575.
- Farahani F.V., Karwowski W., Lighthall N.R. Application of graph theory for identifying connectivity patterns in human brain networks: a systematic review. Front Neurosci 2019; 13: 585, https://doi.org/10.3389/fnins.2019.00585.
- Rubinov M., Sporns O. Complex network measures of brain connectivity: uses and interpretations. Neuroimage 2010; 52(3): 1059–1069, https://doi.org/10.1016/j.neuroimage.2009.10.003.
- Watts D.J., Strogatz S.H. Collective dynamics of ‘small-world’ networks. Nature 1998; 393(6684): 440–442, https://doi.org/10.1038/30918.
- Smit D.J.A., Stam C.J., Posthuma D., Boomsma D.I., de Geus E.J.C. Heritability of “small‐world” networks in the brain: a graph theoretical analysis of resting-state EEG functional connectivity. Hum Brain Mapp 2008; 29(12): 1368–1378, https://doi.org/10.1002/hbm.20468.
- Miranda-Dominguez O., Feczko E., Grayson D.S., Walum H., Nigg J.T., Fair D.A. Heritability of the human connectome: a connectotyping study. Netw Neurosci 2018; 2(2): 175–199, https://doi.org/10.1162/netn_a_00029.
- Arnatkeviciute A., Fulcher B.D., Bellgrove M.A., Fornito A. Where the genome meets the connectome: understanding how genes shape human brain connectivity. Neuroimage 2021; 244: 118570, https://doi.org/10.1016/j.neuroimage.2021.118570.
- Micheloyannis S., Pachou E., Stam C.J., Breakspear M., Bitsios P., Vourkas M., Erimaki S., Zervakis M. Small-world networks and disturbed functional connectivity in schizophrenia. Schizophr Res 2006; 87(1–3): 60–66, https://doi.org/10.1016/j.schres.2006.06.028.
- Anokhin A.P. Genetic psychophysiology: advances, problems, and future directions. Int J Psychophysiol 2014; 93(2): 173–197, https://doi.org/10.1016/j.ijpsycho.2014.04.003.
- Mennes M., Kelly C., Zuo X.N., Di Martino A., Biswal B.B., Castellanos F.X., Milham M.P. Inter-individual differences in resting-state functional connectivity predict task-induced BOLD activity. Neuroimage 2010; 50(4): 1690–1701, https://doi.org/10.1016/j.neuroimage.2010.01.002.
- Cole M.W., Yarkoni T., Repovš G., Anticevic A., Braver T.S. Global connectivity of prefrontal cortex predicts cognitive control and intelligence. J Neurosci 2012; 32(26): 8988–8999, https://doi.org/10.1523/jneurosci.0536-12.2012.
- Grellmann C. Combining brain imaging and genetic data using fast and efficient multivariate correlation analysis. The Faculty of Mathematics and Computer Science, University of Leipzig; 2017.
- Vounou M., Janousova E., Wolz R., Stein J.L., Thompson P.M., Rueckert D., Montana G.; Alzheimer’s Disease Neuroimaging Initiative. Sparse reduced-rank regression detects genetic associations with voxel-wise longitudinal phenotypes in Alzheimer’s disease. Neuroimage 2012; 60(1): 700–716, https://doi.org/10.1016/j.neuroimage.2011.12.029.
- Lee P.H., Shatkay H. An integrative scoring system for ranking SNPs by their potential deleterious effects. Bioinformatics 2009; 25(8): 1048–1055, https://doi.org/10.1093/bioinformatics/btp103.
- Vounou M., Nichols T.E., Montana G.; Alzheimer’s Disease Neuroimaging Initiative. Discovering genetic associations with high-dimensional neuroimaging phenotypes: a sparse reduced-rank regression approach. Neuroimage 2010; 53(3): 1147–1159, https://doi.org/10.1016/j.neuroimage.2010.07.002.
- Zhang X., Xie Y., Tang J., Qin W., Liu F., Ding H., Ji Y., Yang B., Zhang P., Li W., Ye Z., Yu C. Dissect relationships between gene co-expression and functional connectivity in human brain. Front Neurosci 2021; 15: 797849, https://doi.org/10.3389/fnins.2021.797849.
- Bycroft C., Freeman C., Petkova D., Band G., Elliott L.T., Sharp K., Motyer A., Vukcevic D., Delaneau O., O’Connell J., Cortes A., Welsh S., Young A., Effingham M., McVean G., Leslie S., Allen N., Donnelly P., Marchini J. The UK Biobank resource with deep phenotyping and genomic data. Nature 2018; 562(7726): 203–209, https://doi.org/10.1038/s41586-018-0579-z.
- Hawrylycz M.J., Lein E.S., Guillozet-Bongaarts A.L., Shen E.H., Ng L., Miller J.A., van de Lagemaat L.N., Smith K.A., Ebbert A., Riley Z.L., Abajian C., Beckmann C.F., Bernard A., Bertagnolli D., Boe A.F., Cartagena P.M., Chakravarty M.M., Chapin M., Chong J., Dalley R.A., David Daly B., Dang C., Datta S., Dee N., Dolbeare T.A., Faber V., Feng D., Fowler D.R., Goldy J., Gregor B.W., Haradon Z., Haynor D.R., Hohmann J.G., Horvath S., Howard R.E., Jeromin A., Jochim J.M., Kinnunen M., Lau C., Lazarz E.T., Lee C., Lemon T.A., Li L., Li Y., Morris J.A., Overly C.C., Parker P.D., Parry S.E., Reding M., Royall J.J., Schulkin J., Sequeira P.A., Slaughterbeck C.R., Smith S.C., Sodt A.J., Sunkin S.M., Swanson B.E., Vawter M.P., Williams D., Wohnoutka P., Zielke H.R., Geschwind D.H., Hof P.R., Smith S.M., Koch C., Grant S.G.N., Jones A.R. An anatomically comprehensive atlas of the adult human brain transcriptome. Nature 2012; 489(7416): 391–399, https://doi.org/10.1038/nature11405.
- Stein J.L., Hua X., Lee S., Ho A.J., Leow A.D., Toga A.W., Saykin A.J., Shen L., Foroud T., Pankratz N., Huentelman M.J., Craig D.W., Gerber J.D., Allen A.N., Corneveaux J.J., Dechairo B.M., Potkin S.G., Weiner M.W., Thompson P.; Alzheimer’s Disease Neuroimaging Initiative. Voxelwise genome-wide association study (vGWAS). Neuroimage 2010; 53(3): 1160–1174, https://doi.org/10.1016/j.neuroimage.2010.02.032.
- Alfaro-Almagro F., Jenkinson M., Bangerter N.K., Andersson J.L.R., Griffanti L., Douaud G., Sotiropoulos S.N., Jbabdi S., Hernandez-Fernandez M., Vallee E., Vidaurre D., Webster M., McCarthy P., Rorden C., Daducci A., Alexander D.C., Zhang H., Dragonu I., Matthews P.M., Miller K.L., Smith S.M. Image processing and quality control for the first 10,000 brain imaging datasets from UK Biobank. Neuroimage 2018; 166: 400–424, https://doi.org/10.1016/j.neuroimage.2017.10.034.
- Joshi A.A., Choi S., Liu Y., Chong M., Sonkar G., Gonzalez-Martinez J., Nair D., Wisnowski J.L., Haldar J.P., Shattuck D.W., Damasio H., Leahy R.M. A hybrid high-resolution anatomical MRI atlas with sub-parcellation of cortical gyri using resting fMRI. J Neurosci Methods 2022; 374: 109566, https://doi.org/10.1016/j.jneumeth.2022.109566.
- Klein A., Tourville J. 101 labeled brain images and a consistent human cortical labeling protocol. Front Neurosci 2012; 6: 171, https://doi.org/10.3389/fnins.2012.00171.
- Salehi M., Greene A.S., Karbasi A., Shen X., Scheinost D., Constable R.T. There is no single functional atlas even for a single individual: functional parcel definitions change with task. Neuroimage 2020; 208: 116366, https://doi.org/10.1016/j.neuroimage.2019.116366.
- Fan L., Li H., Zhuo J., Zhang Y., Wang J., Chen L., Yang Z., Chu C., Xie S., Laird A.R., Fox P.T., Eickhoff S.B., Yu C., Jiang T. The human brainnetome atlas: a new brain atlas based on connectional architecture. Cereb Cortex 2016; 26(8): 3508–3526, https://doi.org/10.1093/cercor/bhw157.
- Zhang Y., Gong X., Yin Z., Cui L., Yang J., Wang P., Zhou Y., Jiang X., Wei S., Wang F., Tang Y. Association between NRGN gene polymorphism and resting-state hippocampal functional connectivity in schizophrenia. BMC Psychiatry 2019; 19(1): 108, https://doi.org/10.1186/s12888-019-2088-5.
- Egan M.F., Goldberg T.E., Kolachana B.S., Callicott J.H., Mazzanti C.M., Straub R.E., Goldman D., Weinberger D.R. Effect of COMT Val108/158 Met genotype on frontal lobe function and risk for schizophrenia. Proc Natl Acad Sci U S A 2001; 98(12): 6917–6922, https://doi.org/10.1073/pnas.111134598.
- Lachman H.M., Nolan K.A., Mohr P., Saito T., Volavka J. Association between catechol O-methyltransferase genotype and violence in schizophrenia and schizoaffective disorder. Am J Psychiatry 1998; 155(6): 835–837.
- Nolan K.A., Bilder R.M., Lachman H.M., Volavka J. Catechol O-methyltransferase Val158 Met polymorphism in schizophrenia: differential effects of Val and Met alleles on cognitive stability and flexibility. Am J Psychiatry 2004; 161: 359–361, https://doi.org/10.1176/appi.ajp.161.2.359.
- Dennis N.A., Need A.C., LaBar K.S., Waters-Metenier S., Cirulli E.T., Kragel J., Goldstein D.B., Cabeza R. COMT Val108/158 Met genotype affects neural but not cognitive processing in healthy individuals. Cereb Cortex 2010; 20(3): 672–683, https://doi.org/10.1093/cercor/bhp132.
- Wagner G.P., Zhang J. The pleiotropic structure of the genotype–phenotype map: the evolvability of complex organisms. Nat Rev Genet 2011; 12(3): 204–213, https://doi.org/10.1038/nrg2949.
- Wagner G.P., Pavlicev M., Cheverud J.M. The road to modularity. Nat Rev Genet 2007; 8(12): 921–931.
- Bertolero M.A., Blevins A.S., Baum G.L., Gur R.C., Gur R.E., Roalf D.R., Satterthwaite T.D., Bassett D.S. The human brain’s network architecture is genetically encoded by modular pleiotropy. arXiv; 2019, https://doi.org/10.48550/arxiv.1905.07606.
- Elliott L.T., Sharp K., Alfaro-Almagro F., Shi S., Miller K.L., Douaud G., Marchini J., Smith S.M. Genome-wide association studies of brain imaging phenotypes in UK Biobank. Nature 2018; 562(7726): 210–216, https://doi.org/10.1038/s41586-018-0571-7.
- Barber A.D., Hegarty C.E., Lindquist M., Karlsgodt K.H. Heritability of functional connectivity in resting state: assessment of the dynamic mean, dynamic variance, and static connectivity across networks. Cereb Cortex 2021; 31(6): 2834–2844, https://doi.org/10.1093/cercor/bhaa391.
- Jun S., Alderson T.H., Altmann A., Sadaghiani S. Dynamic trajectories of connectome state transitions are heritable. Neuroimage 2022; 256: 119274, https://doi.org/10.1016/j.neuroimage.2022.119274.
- Nentwich M., Ai L., Madsen J., Telesford Q.K., Haufe S., Milham M.P., Parra L.C. Functional connectivity of EEG is subject-specific, associated with phenotype, and different from fMRI. Neuroimage 2020; 218: 117001, https://doi.org/10.1016/j.neuroimage.2020.117001.
- Farrer L.A., Cupples L.A., Haines J.L., Hyman B., Kukull W.A., Mayeux R., Myers R.H., Pericak-Vance M.A., Risch N., van Duijn C.M. Effects of age, sex, and ethnicity on the association between apolipoprotein E genotype and Alzheimer disease. A meta-analysis. APOE and Alzheimer Disease Meta Analysis Consortium. JAMA 1997; 278(16): 1349–1356.
- Smith J.D. Apolipoprotein E4: an allele associated with many diseases. Ann Med 2000; 32: 118–127, https://doi.org/10.3109/07853890009011761.
- Filippini N., Rao A., Wetten S., Gibson R.A., Borrie M., Guzman D., Kertesz A., Loy-English I., Williams J., Nichols T., Whitcher B., Matthews P.M. Anatomically-distinct genetic associations of APOE epsilon4 allele load with regional cortical atrophy in Alzheimer’s disease. Neuroimage 2009; 44(3): 724–728, https://doi.org/10.1016/j.neuroimage.2008.10.003.
- Westlye E.T., Lundervold A., Rootwelt H., Lundervold A.J., Westlye L.T. Increased hippocampal default mode synchronization during rest in middle-aged and elderly APOE ε4 carriers: relationships with memory performance. J Neurosci 2011; 31(21): 7775–7783, https://doi.org/10.1523/jneurosci.1230-11.2011.
- Trachtenberg A.J., Filippini N., Ebmeier K.P., Smith S.M., Karpe F., Mackay C.E. The effects of APOE on the functional architecture of the resting brain. Neuroimage 2012; 59(1): 565–572, https://doi.org/10.1016/j.neuroimage.2011.07.059.
- He B., Gorijala P., Xie L., Cao S., Yan J. Gene co-expression changes underlying the functional connectomic alterations in Alzheimer’s disease. BMC Med Genomics 2022; 15(Suppl 2): 92, https://doi.org/10.1186/s12920-022-01244-6.
- Zeev-Wolf M., Levy J., Ebstein R.P., Feldman R. Cumulative risk on oxytocin-pathway genes impairs default mode network connectivity in trauma-exposed youth. Front Endocrinol (Lausanne) 2020; 11: 335, https://doi.org/10.3389/fendo.2020.00335.
- Sinclair B., Hansell N.K., Blokland G.A., Martin N.G., Thompson P.M., Breakspear M., de Zubicaray G.I., Wright M.J., McMahon K.L. Heritability of the network architecture of intrinsic brain functional connectivity. Neuroimage 2015; 121: 243–252, https://doi.org/10.1016/j.neuroimage.2015.07.048.
- Blake J.A., Ziman M.R. Pax genes: regulators of lineage specification and progenitor cell maintenance. Development 2014; 141(4): 737–751, https://doi.org/10.1242/dev.091785.
- Ruiz-Llorente S., Carrillo Santa de Pau E., Sastre-Perona A., Montero-Conde C., Gómez-López G., Fagin J.A., Valencia A., Pisano D.G., Santisteban P. Genome-wide analysis of Pax8 binding provides new insights into thyroid functions. BMC Genomics 2012; 13: 147, https://doi.org/10.1186/1471-2164-13-147.
- Pasca Di Magliano M., Di Lauro R., Zannini M. Pax8 has a key role in thyroid cell differentiation. Proc Natl Acad Sci U S A 2000; 97(24): 13144–13149, https://doi.org/10.1073/pnas.240336397.
- Williams G.R. Neurodevelopmental and neurophysiological actions of thyroid hormone. J Neuroendocrinol 2008; 20: 784–794, https://doi.org/10.1111/j.1365-2826.2008.01733.x.
- Bernal J. Thyroid hormone receptors in brain development and function. Nat Clin Pract Endocrinol Metab 2007; 3(3): 249–259, https://doi.org/10.1038/ncpendmet0424.
- Dannlowski U., Konrad C., Kugel H., Zwitserlood P., Domschke K., Schöning S., Ohrmann P., Bauer J., Pyka M., Hohoff C., Zhang W., Baune B.T., Heindel W., Arolt V., Suslow T. Emotion specific modulation of automatic amygdala responses by 5-HTTLPR genotype. Neuroimage 2010; 53(3): 893–898, https://doi.org/10.1016/j.neuroimage.2009.11.073.
- Vai B., Serretti A., Poletti S., Mascia M., Lorenzi C., Colombo C., Benedetti F. Cortico-limbic functional connectivity mediates the effect of early life stress on suicidality in bipolar depressed 5-HTTLPR*s carriers. J Affect Disord 2020; 263: 420–427, https://doi.org/10.1016/j.jad.2019.11.142.
- Caspi A., Sugden K., Moffitt T.E., Taylor A., Craig I.W., Harrington H., McClay J., Mill J., Martin J., Braithwaite A., Poulton R. Influence of life stress on depression: moderation by a polymorphism in the 5-HTT gene. Science 2003; 301(5631): 386–389, https://doi.org/10.1126/science.1083968.
- Clarke H., Flint J., Attwood A.S., Munafò M.R. Association of the 5-HTTLPR genotype and unipolar depression: a meta-analysis. Psychol Med 2010; 40(11): 1767–1778, https://doi.org/10.1017/s0033291710000516.
- Sharpley C.F., Palanisamy S.K., Glyde N.S., Dillingham P.W., Agnew L.L. An update on the interaction between the serotonin transporter promoter variant (5-HTTLPR), stress and depression, plus an exploration of non-confirming findings. Behav Brain Res 2014; 273: 89–105, https://doi.org/10.1016/j.bbr.2014.07.030.
- Proshina E.A., Savostyanov A.N., Bocharov A.V., Knyazev G.G. Effect of 5-HTTLPR on current source density, connectivity, and topological properties of resting state EEG networks. Brain Res 2018; 1697: 67–75, https://doi.org/10.1016/j.brainres.2018.06.018.
- Munafò M.R., Durrant C., Lewis G., Flint J. Gene X environment interactions at the serotonin transporter locus. Biol Psychiatry 2009; 65(3): 211–219, https://doi.org/10.1016/j.biopsych.2008.06.009.
- Risch N., Herrell R., Lehner T., Liang K.Y., Eaves L., Hoh J., Griem A., Kovacs M., Ott J., Merikangas K.R. Interaction between the serotonin transporter gene (5-HTTLPR), stressful life events, and risk of depression: a meta-analysis. JAMA 2009; 301(23): 2462–2471, https://doi.org/10.1001/jama.2009.878.
- Knyazev G.G., Bazovkina D.V., Savostyanov A.N., Naumenko V.S., Kuznetsova V.B., Proshina E.A. Suppression mediates the effect of 5-HTTLPR by stress interaction on depression. Scand J Psychol 2017; 58(5): 373–378, https://doi.org/10.1111/sjop.12389.
- Jia P., Kao C.F., Kuo P.H., Zhao Z. A comprehensive network and pathway analysis of candidate genes in major depressive disorder. BMC Syst Biol 2011; 5(Suppl 3): S12, https://doi.org/10.1186/1752-0509-5-s3-s12.
- Richiardi J., Altmann A., Milazzo A.C., Chang C., Chakravarty M.M., Banaschewski T., Barker G.J., Bokde A.L., Bromberg U., Büchel C., Conrod P., Fauth-Bühler M., Flor H., Frouin V., Gallinat J., Garavan H., Gowland P., Heinz A., Lemaître H., Mann K.F., Martinot J.L., Nees F., Paus T., Pausova Z., Rietschel M., Robbins T.W., Smolka M.N., Spanagel R., Ströhle A., Schumann G., Hawrylycz M., Poline J.B., Greicius M.D.; IMAGEN consortium. BRAIN NETWORKS. Correlated gene expression supports synchronous activity in brain networks. Science 2015; 348(6240): 1241–1214, https://doi.org/10.1126/science.1255905.
- Pezawas L., Meyer-Lindenberg A., Drabant E.M., Verchinski B.A., Munoz K.E., Kolachana B.S., Egan M.F., Mattay V.S., Hariri A.R., Weinberger D.R. 5-HTTLPR polymorphism impacts human cingulate-amygdala interactions: a genetic susceptibility mechanism for depression. Nat Neurosci 2005; 8(6): 828–834, https://doi.org/10.1038/nn1463.
- Teeuw J., Brouwer R.M., Guimarães J.P.O.F.T., Brandner P., Koenis M.M.G., Swagerman S.C., Verwoert M., Boomsma D.I., Hulshoff Pol H.E. Genetic and environmental influences on functional connectivity within and between canonical cortical resting-state networks throughout adolescent development in boys and girls. Neuroimage 2019; 202: 116073, https://doi.org/10.1016/j.neuroimage.2019.116073.
- Mills B.D., Grayson D.S., Shunmugavel A., Miranda-Dominguez O., Feczko E., Earl E., Neve K.A., Fair D.A. Correlated gene expression and anatomical communication support synchronized brain activity in the mouse functional connectome. J Neurosci 2018; 38(25): 5774–5787, https://doi.org/10.1523/jneurosci.2910-17.2018.
- Vértes P.E., Rittman T., Whitaker K.J., Romero-Garcia R., Váša F., Kitzbichler M.G., Wagstyl K., Fonagy P., Dolan R.J., Jones P.B., Goodyer I.M.; NSPN Consortium; Bullmore E.T. Gene transcription profiles associated with inter-modular hubs and connection distance in human functional magnetic resonance imaging networks. Philos Trans R Soc Lond B Biol Sci 2016; 371(1705): 20150362, https://doi.org/10.1098/rstb.2015.0362.