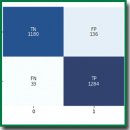
Comparison of an Ensemble of Machine Learning Models and the BERT Language Model for Analysis of Text Descriptions of Brain CT Reports to Determine the Presence of Intracranial Hemorrhage
The aim of this study is to train and test an ensemble of machine learning models, as well as to compare its performance with the BERT language model pre-trained on medical data to perform simple binary classification, i.e., determine the presence/absence of the signs of intracranial hemorrhage (ICH) in brain CT reports.
Materials and Methods. Seven machine learning algorithms and three text vectorization techniques were selected as models to solve the binary classification problem. These models were trained on textual data represented by 3980 brain CT reports from 56 inpatient medical facilities in Moscow. The study utilized three text vectorization techniques: bag of words, TF-IDF, and Word2Vec. The resulting data were then processed by the following machine learning algorithms: decision tree, random forest, logistic regression, nearest neighbors, support vector machines, Catboost, and XGboost. Data analysis and pre-processing were performed using NLTK (Natural Language Toolkit, version 3.6.5), libraries for character-based and statistical processing of natural language, and Scikit-learn (version 0.24.2), a library for machine learning containing tools to tackle classification challenges. MedRuBertTiny2 was taken as a BERT transformer model pre-trained on medical data.
Results. Based on the training and testing outcomes from seven machine learning algorithms, the authors selected three algorithms that yielded the highest metrics (i.e. sensitivity and specificity): CatBoost, logistic regression, and nearest neighbors. The highest metrics were achieved by the bag of words technique. These algorithms were assembled into an ensemble using the stacking technique. The sensitivity and specificity for the validation dataset separated from the original sample were 0.93 and 0.90, respectively. Next, the ensemble and the BERT model were trained on an independent dataset containing 9393 textual radiology reports also divided into training and test sets. Once the ensemble was tested on this dataset, the resulting sensitivity and specificity were 0.92 and 0.90, respectively. The BERT model tested on these data demonstrated a sensitivity of 0.97 and a specificity of 0.90.
Conclusion. When analyzing textual reports of brain CT scans with signs of intracranial hemorrhage, the trained ensemble demonstrated high accuracy metrics. Still, manual quality control of the results is required during its application. The pre-trained BERT transformer model, additionally trained on diagnostic textual reports, demonstrated higher accuracy metrics (p<0.05). The results show promise in terms of finding specific values for both binary classification task and in-depth analysis of unstructured medical information.
- Harrison C.J., Sidey-Gibbons C.J. Machine learning in medicine: a practical introduction to natural language processing. BMC Med Res Methodol 2021; 21(1): 158, https://doi.org/10.1186/s12874-021-01347-1.
- Sheikhalishahi S., Miotto R., Dudley J.T., Lavelli A., Rinaldi F., Osmani V. Natural language processing of clinical notes on chronic diseases: systematic review. JMIR Med Inform 2019; 7(2): e12239, https://doi.org/10.2196/12239.
- Luo J.W., Chong J.J.R. Review of natural language processing in radiology. Neuroimaging Clin N Am 2020; 30(4): 447–458, https://doi.org/10.1016/j.nic.2020.08.001.
- Smorchkova A.K., Khoruzhaya A.N., Kremneva E.I., Petryaikin A.V. Machine learning technologies in CT-based diagnostics and classification of intracranial hemorrhages. Voprosy neirokhirurgii imeni N.N. Burdenko 2023; 87(2): 85–91, https://doi.org/10.17116/neiro20238702185.
- Khanbhai M., Anyadi P., Symons J., Flott K., Darzi A., Mayer E. Applying natural language processing and machine learning techniques to patient experience feedback: a systematic review. BMJ Health Care Inform 2021; 28(1): e100262, https://doi.org/10.1136/bmjhci-2020-100262.
- Spasic I., Nenadic G. Clinical text data in machine learning: systematic review. JMIR Med Inform 2020; 8(3): e17984, https://doi.org/10.2196/17984.
- Davidson E.M., Poon M.T.C., Casey A., Grivas A., Duma D., Dong H., Suárez-Paniagua V., Grover C., Tobin R., Whalley H., Wu H., Alex B., Whiteley W. The reporting quality of natural language processing studies: systematic review of studies of radiology reports. BMC Med Imaging 2021; 21(1): 142, https://doi.org/10.1186/s12880-021-00671-8.
- Gordon A.J., Banerjee I., Block J., Winstead-Derlega C., Wilson J.G., Mitarai T., Jarrett M., Sanyal J., Rubin D.L., Wintermark M., Kohn M.A. Natural language processing of head CT reports to identify intracranial mass effect: CTIME algorithm. Am J Emerg Med 2022; 51: 388–392, https://doi.org/10.1016/j.ajem.2021.11.001.
- Horng H., Steinkamp J., Kahn C.E. Jr., Cook T.S. Ensemble approaches to recognize protected health information in radiology reports. J Digit Imaging 2022; 35(6): 1694–1698, https://doi.org/10.1007/s10278-022-00673-0.
- Tutubalina E., Alimova I., Miftahutdinov Z., Sakhovskiy A., Malykh V., Nikolenko S. The Russian Drug Reaction Corpus and neural models for drug reactions and effectiveness detection in user reviews. Bioinformatics 2021; 37(2): 243–249, https://doi.org/10.1093/bioinformatics/btaa675.
- Devlin J., Chang M.W., Lee K., Toutanova K. BERT: pre-training of deep bidirectional transformers for language understanding. In: Proceedings of the 2019 Conference of the North American Chapter of the Association for Computational Linguistics: Human Language Technologies, Vol. 1. Minneapolis, Minnesota: Association for Computational Linguistics; 2019; p. 4171–4186, https://doi.org/10.48550/arxiv.1810.04805.
- Li J., Lin Y., Zhao P., Liu W., Cai L., Sun J., Zhao L., Yang Z., Song H., Lv H., Wang Z. Automatic text classification of actionable radiology reports of tinnitus patients using bidirectional encoder representations from transformer (BERT) and in-domain pre-training (IDPT). BMC Med Inform Decis Mak 2022; 22(1): 200, https://doi.org/10.1186/s12911-022-01946-y.
- Khadhraoui M., Bellaaj H., Ammar M.B., Hamam H., Jmaiel M. Survey of BERT-base models for scientific text classification: COVID-19 case study. Appl Sci 2022; 12(6): 2891, https://doi.org/10.3390/app12062891.
- Polishchuk N.S., Vetsheva N.N., Kosarin S.P., Morozov S.P., Kuz’mina E.S. Unified radiological information service as a key element of organizational and methodical work of Research and practical center of medical radiology. Radiologia — praktika 2018; 1: 6–17.
- Khoruzhaya А.N., Kozlov D.V., Arzamasov К.M., Kremneva E.I. Text analysis of radiology reports with signs of intracranial hemorrhage on brain CT scans using the decision tree algorithm. Sovremennye tehnologii v medicine 2022; 14(6): 34, https://doi.org/10.17691/stm2022.14.6.04.
- Warner J.L., Levy M.A., Neuss M.N., Warner J.L., Levy M.A., Neuss M.N. ReCAP: feasibility and accuracy of extracting cancer stage information from narrative electronic health record data. J Oncol Pract 2016; 12(2): 157–158, https://doi.org/10.1200/jop.2015.004622.
- Model DmitryPogrebnoy/MedRuBertTiny2. URL: https://huggingface.co/DmitryPogrebnoy/MedRuBertTiny2.
- Hostettler I.C., Muroi C., Richter J.K., Schmid J., Neidert M.C., Seule M., Boss O., Pangalu A., Germans M.R., Keller E. Decision tree analysis in subarachnoid hemorrhage: prediction of outcome parameters during the course of aneurysmal subarachnoid hemorrhage using decision tree analysis. J Neurosurg 2018; 129(6): 1499–1510, https://doi.org/10.3171/2017.7.jns17677.
- Improving BERT-based model for medical text classification with an optimization algorithm. In: Advances in computational collective intelligence. ICCCI 2022. Communications in computer and information science, vol. 1653. Bădică C., Treur J., Benslimane D., Hnatkowska B., Krótkiewicz M. (editors). Springer, Cham; 2022, https://doi.org/10.1007/978-3-031-16210-7_8.
- Taghizadeh N., Doostmohammadi E., Seifossadat E., Rabiee H.R., Tahaei M.S. SINA-BERT: a pre-trained language model for analysis of medical texts in Persian. arXiv; 2021, https://doi.org/10.48550/arxiv.2104.07613.
- Bressem K.K., Papaioannou J.M., Grundmann P., Borchert F., Adams L.C., Liu L., Busch F., Xu L., Loyen J.P., Niehues S.M., Augustin M., Grosser L., Makowski M.R., Aerts H.J.W.L., Löser A. MEDBERT.de: a comprehensive German BERT model for the medical domain. arXiv; 2023, https://doi.org/10.48550/arxiv.2303.08179.
- Çelıkten A., Bulut H. Turkish medical text classification using BERT. In: 29th Signal Processing and Communications Applications Conference (SIU). Istanbul; 2021; p. 1–4, https://doi.org/10.1109/siu53274.2021.9477847.
- Kim Y., Kim J.H., Lee J.M., Jang M.J., Yum Y.J., Kim S., Shin U., Kim Y.M., Joo H.J., Song S. A pre-trained BERT for Korean medical natural language processing. Sci Rep 2022; 12(1): 13847, https://doi.org/10.1038/s41598-022-17806-8.
- Xue K., Zhou Y., Ma Z., Ruan T., Zhang H., He P. Fine-tuning BERT for joint entity and relation extraction in Chinese medical text. In: 2019 IEEE International Conference on Bioinformatics and Biomedicine (BIBM). San Diego, CA; 2019; p. 892–897, https://doi.org/10.1109/bibm47256.2019.8983370.
- Wu Z., Liang J., Zhang Z., Lei J. Exploration of text matching methods in Chinese disease Q&A systems: a method using ensemble based on BERT and boosted tree models. J Biomed Inform 2021; 115: 103683, https://doi.org/10.1016/j.jbi.2021.103683.
- Pavlov N.A., Andreychenko A.E., Vladzymyrskyy A.V., Revazyan A.A., Kirpichev Y.S., Morozov S.P. Reference medical datasets (MosMedData) for independent external evaluation of algorithms based on artificial intelligence in diagnostics. Digital diagnostics 2021; 2(1): 49–66, https://doi.org/10.17816/dd60635.
- Vladzimirsky A.V., Gusev A.V., Sharova D.E., Shulkin I.M., Popov A.A., Balashov M.K., Omelyanskaya O.V., Vasilyev Y.A. Health information system maturity assessment methodology. Vrac i informacionnye tehnologii 2022; 3: 68–84, https://doi.org/10.25881/18110193_2022_3_68.